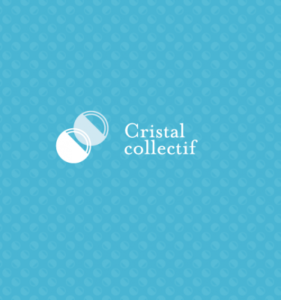
Séminaire
Bridging observation, theory and numerical simulation of the ocean using Machine Learning
This talk aims at showing some of the recent advancements in using Machine Learning for ocean modeling, it is also an opportunity to discuss the promising research avenues that can bring together ML researchers and ocean modelers.
Description
This talk aims at showing some of the recent advancements in using Machine Learning for ocean modeling, it is also an opportunity to discuss the promising research avenues that can bring together ML researchers and ocean modelers. Two applications are highlighted:
Clustering and Tracking Ocean Regimes using transparent machine learning: The North Atlantic ocean is key to climate through its role in heat transport and storage, but the response of the circulation’s drivers to a changing climate is poorly constrained. The transparent machine learning method Tracking global Heating with Ocean Regimes (THOR) identifies drivers of circulation with minimal input: depth, dynamic sea level and wind stress. Beyond a black box approach, THOR’s predictive skill is transparent. A dataset is created with features engineered and labeled by an explicitly interpretable equation transform and k-means application. A multilayer perceptron is then trained, explaining its skill using relevance maps and theory. THOR reveals a weakened circulation with abrupt CO2 quadrupling, due to a shift in deep water formation areas and locations of the Gulf Stream and Trans Atlantic Current transporting heat northward. If CO2 is increased 1% yearly, similar but transient patterns emerge. THOR could accelerate model analysis and facilitate process oriented intercomparisons.
History Matching for the tuning of oceanic models, a study case on the Lorenz96 model: Here I’ll present a tool from the Uncertainty Quantification community that started recently to draw attention in climate modeling: History Matching also referred to as « Iterative Refocussing ». The core idea of History Matching is to run several simulations with different set of parameters and then use observed data to rule-out any parameter settings which are « implausible ». Since climate simulation models are computationally heavy and do not allow testing every possible parameter setting, we employ an emulator that can be a cheap and accurate replacement. Here a machine learning algorithm, namely, Gaussian Process Regression is used for the emulating step. History Matching is then a good example where the recent advances in machine learning can be of high interest to climate modeling. I will show some results using History Matching on a toy model: the two-layer Lorenz96, and share some findings about the challenges and opportunities of using this technique.
Informations supplémentaires
Séminaire en ligne : https://global.gotomeeting.com/join/349269413